The 3rd Generation of Financial Planning
4 September 2020
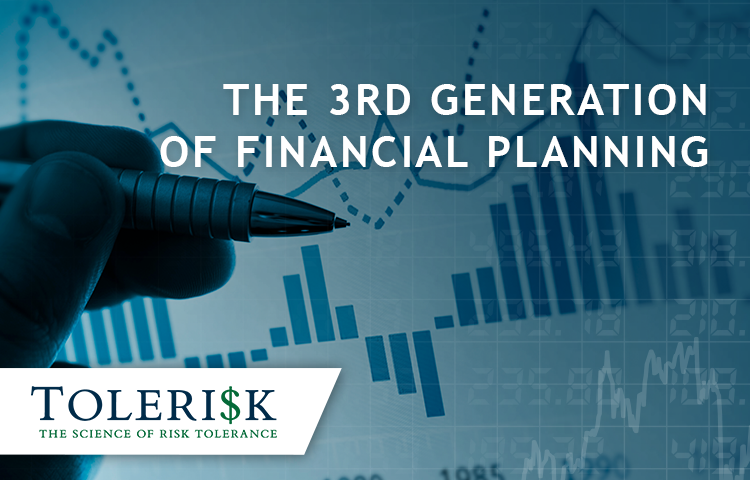
Abstract
The first generation of financial planning (1970s through 1990s) used static modeling with a constant return expectation. The chief limitation was that it didn’t accurately reflect the real sequence of returns observed, which created very unrealistic results and underrepresented the risk of failure. In this century, financial plans were primarily conducted using single-factor Monte Carlo simulations (the second generation of financial planning), which addressed the sequence of return risk. While a vast improvement over the first generation of financial plans, typical Monte Carlo simulations still have material weaknesses in how they represent potential future circumstances. The most notable weaknesses include the assumption of a constant risk level (determining expected return), a constant volatility (standard deviation) for potential portfolio returns, a single path for potential inflation and a constant terminal point for evaluating the simulation. The third generation of financial planning mathematical infrastructure has emerged, pioneered by Tolerisk (2012), which incorporates additional variables to more realistically reflect potential future outcomes.
The First Generation – Static Models
As computing power evolved, the ability to incorporate more intricate cash-flow assumptions improved. General assumptions for portfolio returns, taxes and inflation expectations evolved. Even life expectancy changed and thus the time horizon for the financial plan increased. These improved assumptions enhanced the reliability of the outcome. However, they were often biased based on the then current economic climate (e.g. late 1970s/early 1980s with inflation and interest rates above 10%), which didn’t necessarily accurately reflect an uncertain future.
The chief limitation of the first generation of financial plans was inherent in the portfolio return assumptions. An individual could be lucky enough to retire at the beginning of a strong market or unlucky enough to retire at the beginning of a weak market economy. The difference could be as stark as success or failure. Assuming, for example, a 6% return every year ignored the massive variance that occurred during most retirement horizons.
The Second Generation – Monte Carlo Simulation
For the last two decades, financial planning systems have been dominated by Monte Carlo simulations. These generally run 1,000 or more randomly generated paths of potential portfolio returns. They are usually calibrated to a single volatility (standard deviation) and expected-return assumption, both driven by the user-specified risk level of the portfolio. These simulations generally hold the risk level constant, although some allow a subjective change at a specific time in the future. Practitioners commonly assume a singular reduction in portfolio risk directive upon retirement. While the typical financial planning Monte Carlo simulation randomly generates potential return paths, it is most common for them to utilize a single path for future inflation. This may be customized by the distribution of expenses for the client and may change over time as the composition of expenses evolves. These generally do not vary with each potential path of portfolio returns in the simulation.
Monte Carlo simulations are a big advancement compared to static models, primarily because they address the sequence of return risk prevalent in financial plans. However, Monte Carlo simulations used in financial planning models have a number of common limitations and short-falls when considering the range of potential realities of a person’s financial future.
The fact that financial plans can often be 50+ years for younger clients inherently makes some of today’s Monte Carlo assumptions unreflective of the realities of a long-term future. The notion that the volatility of the stock market (or bond market or inflation) would be constant for 50 or 60 years isn’t appealing when considering how varied volatility has been historically. A similar weakness exists when contemplating the constant correlation between stocks and bonds for 50+ years. Simply the fact that inflation is generally modeled as a single path and doesn’t vary reduces the reliability of the simulation results. One shouldn’t assume that risk and mortality are constant either, given the uncertainty of tomorrow’s reality.
Alternate 2nd Generation – Multiple Static Scenarios
Although not as popular, during the same time period that Monte Carlo simulations took off, some advisors and planners began running multiple static scenarios that incorporated portfolio return variance as observed historically. Frequently 30-year periods were considered as a representation of a realistic retirement period. Some plans used rolling 30-year periods, where there might exist 60 rolling 30-year periods in a 90-year history. The criticism of this approach is that the middle 30 years amidst the 90-year history would be counted more than the first 30 years or the most recent 30 years. This disproportionate weight to the center of the data set used, combined with the limitation of a small number of potential paths (e.g. 60 rolling 30-year periods), may have prevented this approach from garnering more interest, as it was overshadowed by Monte Carlo simulation models.
The Third Generation
Cutting-edge financial planning mathematics remediates the key weaknesses in the application of the typical Monte Carlo simulation in financial plans. It utilizes long-dated and detailed historical data to create (nearly) infinite paths without the use of random numbers. The 1,000+ paths have historical frequency and relevance without the limitation of over- or under-weighted historical periods. It utilizes separate paths for equities, fixed income, and inflation. This process also incorporates the dynamic volatility and dynamic correlations between each data series. By employing an objective measurement of someone’s ability to take risk, an evolving custom glidepath for risk in the future can be incorporated into all aspects of the analysis. Further, this process layers in customized, last-survivor probabilities by year into the simulation.
By adding an evolving risk directive and dynamic inflation and mortality to the simulation process, a more robust and realistic representation of potential future outcomes is achieved. Here are the key aspects of this dynamic modeling:
Risk directive – By measuring the mathematical ability (capacity) to take risk now and in the future, a dynamic, customized glide path for risk in the future can be incorporated into all aspects of the financial plan and its analysis. Most advisors recognize that today’s risk level is not necessarily the most likely indication of the client’s risk level in the future. The appropriate glidepath for risk will likely evolve as the client migrates towards and through their future cash-flows.
Inflation – Using a separate set of 1,000+ paths for inflation (CPI), the sequence of real (inflation-adjusted) return risk is addressed. There may be periods of time when returns are generally average but inflation is materially above expectation, which can compromise a plan because higher future costs would deplete future asset values. Conversely, there may be periods of below average portfolio returns that are accompanied by inflation that is below expectation, which may not compromise someone’s financial longevity. The sequence of real returns is a more realistic contributor to financial planning success than simply the sequence of nominal return risk.
Volatility – Using paths with evolving volatility better represent the observable history in stocks, bonds, and inflation. Even over extended periods of time, we note significant historical variance of volatility itself.
Correlation – By using separate paths for stocks, bonds, and inflation, each of which with dynamic volatility, we inherently create variable correlation between these series as it occurred historically. In reality, we observe that correlations between stocks, bonds, and inflation can change materially, even over long periods of time.
Mortality –Recognizing and incorporating the variable mortality both individually and amongst a last-surviving spouse improves the validity of measuring the probability of failure. It also eliminates the need to exaggerate the static terminus to accommodate the increased probability that at least one individual within a couple will survive to an advanced age.
Conclusion
By introducing additional variables to the simulation process within a financial plan, a more realistic and reliable representation of probable outcomes will be achieved. The third generation of financial planning incorporates dynamic correlations between stocks, bonds, and inflation as well as dynamic volatilities of each data series. It incorporates dynamic risk directive expectations that evolve as clients migrate towards and through their cash-flow series. Layering into the simulation process the customizable, last-survivor probabilities per annum facilitates an encompassing probability of outliving one’s money, currently the most robust metric in planning technology.
This latest generation mathematical infrastructure is now available broadly through API integration, licensed by Tolerisk. It can be utilized by financial planning software, robo-advice solutions, recordkeeping technology, and more. If you are interested in learning more about how Tolerisk’s next generation mathematical infrastructure can elevate your financial services application, please contact us: integrations@tolerisk.com.
Mark Friedenthal is founder and CEO of Tolerisk, a cloud-based SAAS tool for financial advisors to provide better risk tolerance assessments for their clients.